Spotlight on Vanderbilt Biostatistics: Shawn Garbett
This week we are excited to feature one of our IT team members in the Spotlight series. Shawn Garbett, a Senior Application Developer in the Vanderbilt Department of Biostatistics, tells us more about his interests and research.
What was your draw to statistics and/or Vanderbilt?
I have always had a love of mathematical modeling throughout my career starting with hydrology models at TVA. I was hired into the Quaranta lab doing mathematical models of cancer progression here at Vanderbilt and got to work with a wonderful group. There was a desire to tie the models to observed microscopy data and I discovered the depth of statistical reasoning researching the problem. This led to statistics becoming a new passionate pursuit to the point I went back and got my masters at Penn State in applied statistics. Working with a department full of world class statisticians is a dream come true.
Tell us about a major finding in your current research.
On my current health policy project, I've found a technique that allows one to have tunnel states with memory accurately inside a Markov model. Many observed risks are of the form "For X years after event Y the risk is Z". This violates Markov assumptions as it requires memory of an event in time, but Markov models are a preferred method in the economic modeling domain. With this method discovery one can continue to the Markov modeling framework with minor adaptation without relying on ad hoc methods while having states with memory.
Tell us about a significant publication that you've contributed to during your career so far.
If I were to pick a publication to highlight, it's "An unbiased metric of antiproliferative drug effect in vitro". Mass screenings of cancer drugs were based on a metric developed back in the 1970s. The growth inhibition effects were being estimated without log scaling the observed cell sizes. Further complicating the observations is that there is a non-linear (even on log scale) effect as cell requilibriate to their new conditions, i.e. long term response is not short term response. A more robust measurement of growth effect could be made with the same equipment, but by simply log scaling data and shifting the time window of observation post equilibration. This has led to increased accuracy in cancer drug screening.
What is your philosophy about teaching and/or research, and what is your best advice for aspiring statisticians?
I think teaching and research go hand in hand, and love the fact that statistics is now part of elementary education in the US. Sarah Fletcher was a summer student in the Quaranta lab and I mentored her at the time. She wanted to really make the microscopy estimates we had as robust as possible and she educated me on how statistical reasoning was the best approach to tying modeling to microscope observations. I introduced her to Jeffery Blume and she went on to be one the first graduate biostatistics students here at Vanderbilt. She's now at Harvard. I was lucky to have her as a student.
I think the best advice for aspiring statisticians is to question every assumption. Make sure that one knows every assumption of the techniques being used, test them, and explore them.
What are your thoughts on controversial statistical topics such as the role of data science in the future, adjusting the p-value for multiple comparison, the choice between Bayesian, likelihood, or frequentists, ethical issues, etc.?
I think data science runs the risk of mass "plug and chug" approaches from folks who are not aware of the pitfalls of analyzing observation data. A majority of the really large data sets available in data science are observational. This is a big opportunity for statisticians because they've had years of research into this very topic and have many tools for dealing with it. I heard recently the term 'multiple regression' at a data science symposium referred to as 'artificial intelligence' multiple times.
Statisticians have worked with this AI since the 1920's! The value of statistical reasoning is now being realized on a global scale. I predict that over time the distinction between observational and random data will get highlighted far earlier in education, even possibly in introductory courses to address this issue.
I personally am attracted to likelihood methods in general, and like the idea that a p-test shouldn't necessarily be against a point estimate but a relevant set. On a related topic, the term "significant" has a very different meaning to those outside the field and is a continued barrier in communicating to a broader public. I like to use "discernible" as a substitute when communicating to a lay audience. Communication of statistical results is going to be increasingly important for rational choices in the public sector.
Tell us about your life outside of Vanderbilt. Do you have a significant other? Children? Pets? Hobbies?
I am married to Lisa McCawley in the Department of Biomedical Engineering at Vanderbilt. I have 2 daughters, one attending Ohio Wesleyan and the other at Hume Fogg. We also have 2 cats. I love to play strategic board games and play piano, and on a cold cloudless night I can be found in a field looking through a telescope.
Finally, what is something about you that most people at Vanderbilt still don't know about you? (Until now, of course!)
I worked for many years doing formal models of software development for the government and on one contract wrote the control software for an infusion pump for emergency bullet wound treatment. It was the first closed loop device ever approved by the FDA. I didn't know at the time, but the client was the White House and the first deliveries all went to Air Force One.
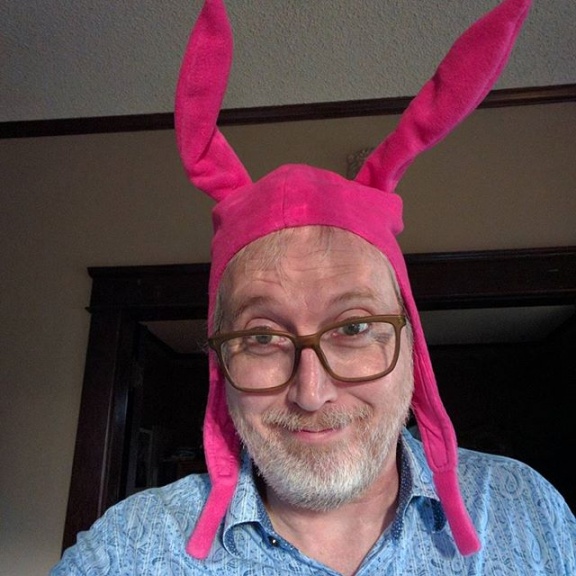